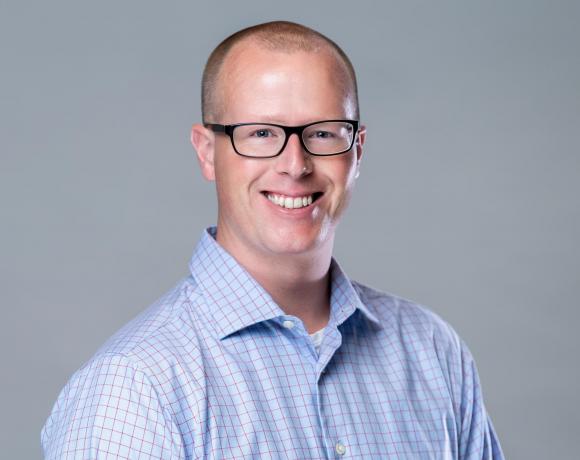
Roth, Andrew
PhD
Academic Rank(s): Assistant Professor, Pathology and Laboratory Medicine, UBC | BCCA, Department of Molecular Oncology | PI, UBC Department of Computer Science
Affiliation(s): BC Cancer Research Centre
Research and Scholarly Interests: Cancer, Informatics
Clinical Interests:
Dr. Andrew Roth, PhD (UBC) is an assistant professor in the Departments of Pathology and Laboratory Medicine, and Computer Science. His interdisciplinary research focuses on the application of statistical machine learning to problems in high dimensional cancer biology. This research program encompasses the development of computational methods for studying clonal population structures and tumour evolution, as well as methodological work in computational statistics. His work in computational biology has been published in Nature Methods and applied in high profile studies of breast and ovarian cancer published in Nature and Nature Genetics. Dr. Roth will provide expertise in bioinformatics analysis. In particular, he will focus on developing computational approaches for studying the spatial and temporal dynamics of the malignant-cell immune interface.
Academic Background
- PhD. Bioinformatics, UBC. 2015
- BSc with Hons. University of British Columbia, Departments of Physics and Mathematics. 2009
Awards and Recognition
- 2013 Canadian Institutes of Health Research – Frederick Banting and Charles Best Canada Graduate Scholarships Doctoral Award
- 2009 Natural Sciences and Engineering Research Council of Canada – Alexander Graham Bell Canada Graduate Scholarship Masters Award
- 2009 Canadian Institutes of Health Research – Bioinformatics Training Program Scholarship
- 2008 Natural Sciences and Engineering Research Council of Canada – Undergraduate Student Research Award
Publications
Selected Publications
Research Interest
My research is primarily focused on the development and application of statistical and machine learning methods for analysing cancer omics data. The methods I develop leverage probabilistic graphical models and non-parametric Bayesian methods to extract biologically interpretable quantities from complex datasets. I am also interested in developing computationally efficient inference algorithms to fit these models. I am particularly interested in variational and sequential Monte Carlo methods. These two themes support the ultimate goal of my research which is to understand evolutionary cancer biology.
Clinical Service
Current Projects In My Lab Include
Teaching Interest